Posted by Crime Tech Solutions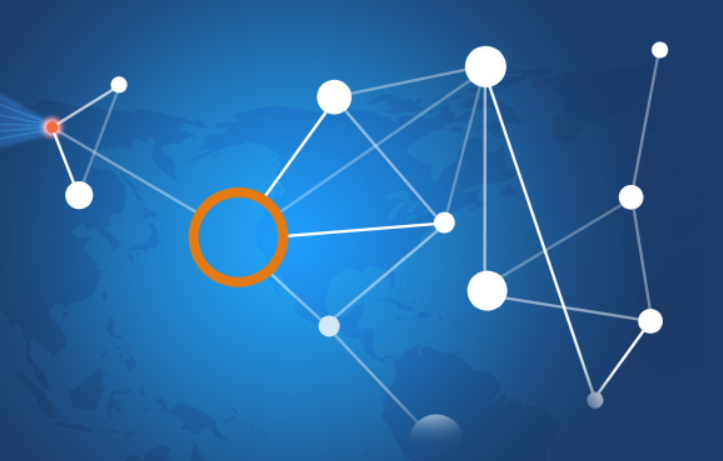
Some linkage data, such as telephone call detail records, may be simple but voluminous, with uniform node and link types and a great deal of regularity. Other data, such as law enforcement data, may be extremely rich and varied, though sparse, with elements possessing many attributes and confidence values that may change over time.
Various techniques are appropriate for distinct problems. For example, heuristic, localized methods might be appropriate for matching known patterns to a network of financial transactions in a criminal investigation. Efficient global search strategies, on the other hand, might be best for finding centrality or severability in a telephone network.
Link analysis can be broken down into two components—link generation, and utilization of the resulting linkage graph.
Link Generation
Link generation is the process of computing the links, link attributes and node attributes. There are several different ways to define links. The different approaches yield very different linkage graphs. A key aspect in defining a link analysis is deciding which representation to use.
Explicit Links
A link may be created between the nodes corresponding to each pair of entities in a transaction. For example, with a call detail record, a link is created between the originating telephone number and the destination telephone number. This is referred to as an explicit link.
Aggregate Links
A single link may be created from multiple transactions. For example, a single link could represent all telephone calls between two parties, and a link attribute might be the number of calls represented. Thus, several explicit links may be collapsed into a single aggregate link.
Inferred Relationships
Links may also be created between pairs of nodes based on inferred strengths of relationships between them. These are sometimes referred to as soft links, association links, or co-occurrence links. Classes of algorithms for these computations include association rules, Bayesian belief networks and context vectors. For example, a link may be created between any pair of nodes whose context vectors lie within a certain radius of one another. Typically, one attribute of such a link is the strength of the relationship it represents. Time is a key feature that offers an opportunity to uncover linkages that might be missed by more typical data analysis approaches. For example, suppose a temporal analysis of wire transfer records indicates that a transfer from account A to person X at one bank is temporally proximate to a transfer from account B to person Y at another bank. This yields an inferred link between accounts A and B. If other aspects of the accounts or transactions are also suspicious, they may be flagged for additional scrutiny for possible money laundering activity.
A specific instance of inferred relationships is identifying two nodes that may actually correspond to the same physical entity, such as a person or an account. Link analysis includes mechanisms for collapsing these to a single node. Typically, the analyst creates rules or selects parameters specifying in which instances to merge nodes in this fashion.
Utilization
Once a linkage graph, including the link and node attributes, has been defined, it can be browsed, searched or used to create variables as inputs to a decision system.
Visualization
In visualizing linking graphs, each node is represented as an icon, and each link is represented as a line or an arrow between two nodes. The node and link attributes may be displayed next to the items or accessed via mouse actions. Different icon types represent different entity types. Similarly, link attributes determine the link representation (line strength, line color, arrowhead, etc.).
Standard graphs include spoke and wheel, peacock, group, hierarchy and mesh. An analytic component of the visualization is the automatic positioning of the nodes on the screen, i.e., the projection of the graph onto a plane. Different algorithms position the nodes based on the strength of the links between nodes or to agglomerate the nodes into groups of the same kind. Once displayed, the user typically has the ability to move nodes, modify node and link attributes, zoom in, collapse, highlight, hide or delete portions of the graph.
Variable Creation
Link analysis can append new fields to existing records or create entirely new data sets for subsequent modeling stages in a decision system. For example, a new variable for a customer might be the total number of email addresses and credit card numbers linked to that customer.
Search
Link analysis query mechanisms include retrieving nodes and links matching specified criteria, such as node and link attributes, as well as search by example to find more nodes that are similar to the specified example node.
A more complex task is similarity search, also called clustering. Here, the objective is to find groups of similar nodes. These may actually be multiple instances of the same physical entity, such as a single individual using multiple accounts in a similar fashion.
Network Analysis
Network analysis is the search for parts of the linkage graph that play particular roles. It is used to build more robust communication networks and to combat organized crime. This exploration revolves around questions such as:
- Which nodes are key or central to the network?
- Which links can be severed or strengthened to most effectively impede or enhance the operation of the network?
- Can the existence of undetected links or nodes be inferred from the known data?
- Are there similarities in the structure of subparts of the network that can indicate an underlying relationship (e.g., modus operandi)?
- What are the relevant sub-networks within a much larger network?
- What data model and level of aggregation best reveal certain types of links and sub-networks?
- What types of structured groups of entities occur in the data set?
Applications
Link analysis tools such as those provided by Crime Tech Solutions are increasingly used in law enforcement investigations, detecting terrorist threats, fraud detection, detecting money laundering, telecommunications network analysis, classifying web pages, analyzing transportation routes, pharmaceuticals research, epidemiology, detecting nuclear proliferation and a host of other specialized applications. For example, in the case of money laundering, the entities might include people, bank accounts and businesses, and the transactions might include wire transfers, checks and cash deposits. Exploring relationships among these different objects helps expose networks of activity, both legal and illegal.